Revolutionizing Financial Services:
AI-Driven Loan Approval Process Optimization
Project Goal
To implement an AI-powered loan approval system that streamlines the application process, reduces processing time, and improves risk assessment accuracy while maintaining regulatory compliance.
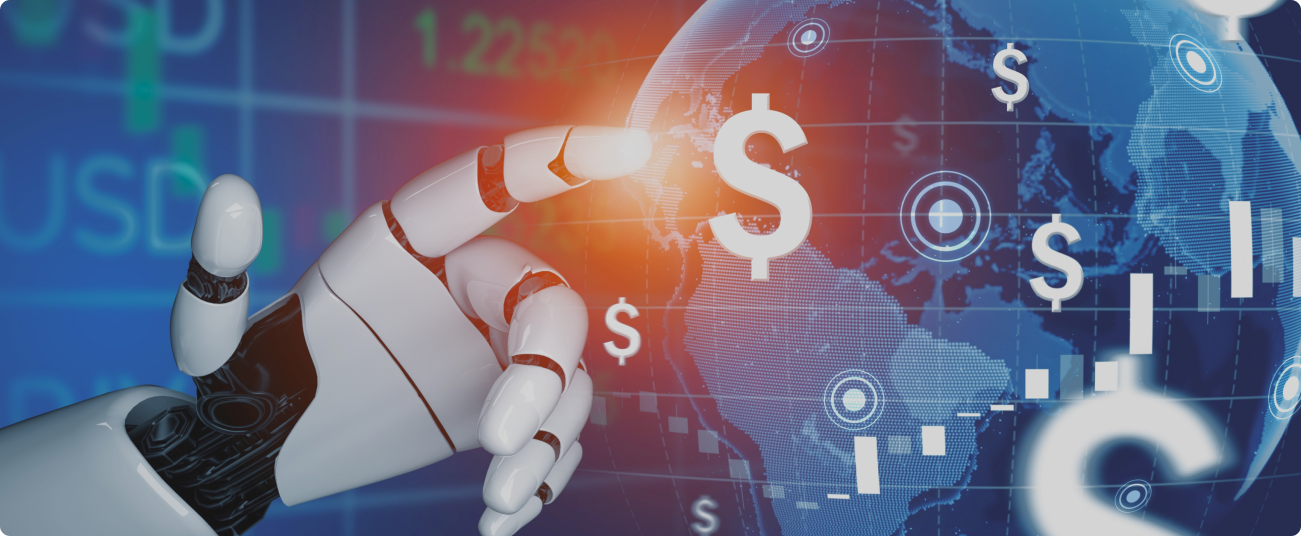
Industry
Financial Technology (Fintech)
Location
New York City, USA
Tech Team
Project Manager | Data Scientists | ML Engineers | Risk Assessment Specialists | UX Designer | Full Stack Engineers (React/Python) | Compliance Officers
Team Involved
- Project manager
- Data Scientists
- ML Engineers
- Risk Assessment Specialists
- Full Stack Engineers (React/Python)
- Compliance Officers
- UX Designer
Business Tasks the Client Wanted to Solve
Accelerate Loan Processing
- Reduce the time taken to process and approve loan applications through automation of manual review processes
- Enable real-time preliminary loan decisions
Enhance Risk Assessment
- Implement more sophisticated risk assessment models using multiple data points
- Improve the accuracy of default prediction
- Reduce human bias in the loan approval process
Change Any Particular Thing in the Generated Image
- Handle increasing application volumes without proportionally increasing staff
- Maintain consistency in loan evaluations across all applications
- Enable simultaneous processing of multiple applications.
Ensure Compliance
- Maintain transparent decision-making processes
- Provide clear audit trails for regulatory requirements
- Implement fair lending practices
Improve Customer Experience
- Reduce waiting times for loan decisions
- Provide clear feedback on application status
- Enable digital document submission and verification
Business Tasks the Client Wanted to Solve:
1) Accelerate Loan Processing
- Reduce the time taken to process and approve loan applications through automation of manual review processes
- Enable real-time preliminary loan decisions
2) Enhance Risk Assessment
- Implement more sophisticated risk assessment models using multiple data points
- Improve the accuracy of default prediction
- Reduce human bias in the loan approval process
3) Change Any Particular Thing in the Generated Image
- Handle increasing application volumes without proportionally increasing staff
- Maintain consistency in loan evaluations across all applications
- Enable simultaneous processing of multiple applications.
4) Ensure Compliance
- Maintain transparent decision-making processes
- Provide clear audit trails for regulatory requirements
Implement fair lending practices
5 ) Improve Customer Experience
- Reduce waiting times for loan decisions
- Provide clear feedback on application status
- Enable digital document submission and verification
What pitfalls did the client face?

Legacy System Integration
Existing systems were not designed for AI integration. Historical data was stored in various formats and locations. Manual processes were deeply embedded in operations.

Data Quality & Standardization
Inconsistent data formats across different sources. Missing or incomplete historical data. Lack of standardized documentation processes.

Staff Resistance
Concerns about job security. Reluctance to adopt new technologies. Learning curve for new systems

Regulatory Compliance
Need for explainable AI decisions. Ensuring fair lending practices. Meeting data privacy requirements.
What pitfalls did the client face?
1) Legacy System Integration
- Existing systems were not designed for AI integration
- Historical data was stored in various formats and locations
- Manual processes were deeply embedded in operations
2) Data Quality and Standardization
- Inconsistent data formats across different sources
- Missing or incomplete historical data
- Lack of standardized documentation processes.
3) Regulatory Compliance
- Need for explainable AI decisions
- Ensuring fair lending practices
- Meeting data privacy requirements
4) Staff Resistance
- Concerns about job security
- Reluctance to adopt new technologies
- Learning curve for new systems
Our Suggestions
Initial Assessment and Planning
- Conduct thorough analysis of existing loan approval processes
- Identify key pain points and automation opportunities
- Define success metrics and compliance requirements
- Create implementation roadmap
System Design and Architecture
- Backend: Use Python and TensorFlow for AI model development. Leverage cloud services for scalability and data management.
- Frontend: React-based dashboard for loan officers
- Database: MongoDB for flexible data storage
- API Layer: FastAPI for high-performance backend services
Development and Integration
- Implement modular AI components for different aspects of loan processing
- Create explainable AI features for transparency
- Develop real-time monitoring and alerting systems
- Build robust data validation and cleaning pipelines
Testing and Deployment
- Conduct parallel testing with existing systems
- Implement gradual rollout strategy
- Provide comprehensive staff training
- Establish feedback loops for continuous improvement
What we suggested:
1. Initial Assessment and Planning
- Conduct thorough analysis of existing loan approval processes
- Identify key pain points and automation opportunities
- Define success metrics and compliance requirements
- Create implementation roadmap
2. System Design and Architecture
- Backend: Use Python and TensorFlow for AI model development. Leverage cloud services for scalability and data management.
- Frontend: React-based dashboard for loan officers
- Database: MongoDB for flexible data storage
- API Layer: FastAPI for high-performance backend services
3. Development and Integration
- Implement modular AI components for different aspects of loan processing
- Create explainable AI features for transparency
- Develop real-time monitoring and alerting systems
- Build robust data validation and cleaning pipelines
4. Testing and Deployment
- Conduct parallel testing with existing systems
- Implement gradual rollout strategy
- Provide comprehensive staff training
- Establish feedback loops for continuous improvement
Your Business Could Be the Next Success Story
We turn complex challenges into scalable digital solutions.
Let’s talk about how we can solve yours.
Technical Architecture

AI/ML Stack
- TensorFlow for deep learning models
- scikit-learn for traditional ML algorithms
- XGBoost for gradient boosting
- SHAP for model explainability

Backend Framework
- FastAPI for high-performance API development
- Celery for task queue management
- Redis for caching
- MongoDB for document storage

Frontend Framework
- T React with TypeScript
- Redux for state management
- Material-UI for component library
- D3.js for data visualization

Cloud Services
- AWS ECS for containerized applications
- AWS Lambda for serverless functions
- Amazon S3 for document storage
- Amazon RDS for relational data

Security and Compliance
- AWS KMS for encryption
- OAuth 2.0 for authentication
- Regular security audits
- Automated compliance checking

Monitoring and Analytics
- ELK Stack for log management
- Prometheus for metrics
- Grafana for dashboards
- Custom analytics for model performance
Technical architecture:
1. AI/ML Stack
- TensorFlow for deep learning models
- scikit-learn for traditional ML algorithms
- XGBoost for gradient boosting
- SHAP for model explainability
2. Backend Framework
- FastAPI for high-performance API development
- Celery for task queue management
- Redis for caching
- MongoDB for document storage
3. Frontend Framework
- T React with TypeScript
- Redux for state management
- Material-UI for component library
- D3.js for data visualization
4. Cloud Services
- AWS ECS for containerized applications
- AWS Lambda for serverless functions
- Amazon S3 for document storage
- Amazon RDS for relational data
5. Security and Compliance
- AWS KMS for encryption
- OAuth 2.0 for authentication
- Regular security audits
- Automated compliance checking
6. Monitoring and Analytics
- ELK Stack for log management
- Prometheus for metrics
- Grafana for dashboards
- Custom analytics for model performance
Business Outcomes
Operational Efficiency
- 40% reduction in loan processing time
- 60% decrease in manual document review
- 85% automation of routine tasks
Risk Management
- 25% improvement in risk assessment accuracy
- 30% reduction in default rates
- Enhanced fraud detection capabilities
Customer Satisfaction
- 50% faster loan decisions
- 70% reduction in application errors
- Improved transparency in decision-making
Scalability
- 3x increase in application processing capacity
- 45% reduction in operational costs
- Improved resource utilization
Compliance and Reporting
- 100% audit trail coverage
- Automated compliance reporting
- Reduced regulatory risks
Business Outcomes:
1. Operational Efficiency
- 40% reduction in loan processing time
- 60% decrease in manual document review
- 85% automation of routine tasks
2. Risk Management
- 25% improvement in risk assessment accuracy
- 30% reduction in default rates
- Enhanced fraud detection capabilities
3. Customer Satisfaction
- 50% faster loan decisions
- 70% reduction in application errors
- Improved transparency in decision-making
4. Scalability
- 3x increase in application processing capacity
- 45% reduction in operational costs
- Improved resource utilization
5. Compliance and Reporting
- 100% audit trail coverage
- Automated compliance reporting
- Reduced regulatory risks